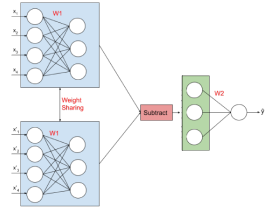
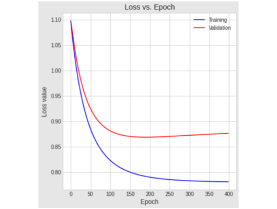
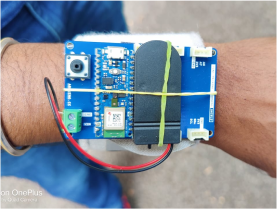
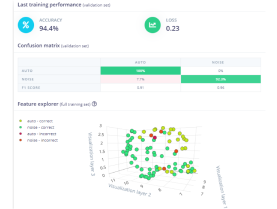
Deep Learning Principles & Applications
Deep learning is a widely and immediately applicable AI tool that is actively used in several disciplines across engineering, natural sciences, health care, finance, and humanities. This workshop aims to provide the participants a hands-on computation-based introduction to the principles and applications of deep learning. Leveraging on a quick, yet accessible introduction to key ideas from linear algebra, participants will be introduced to building a simple neural network from scratch and understanding the fundamental operating principle—the “learning” process. Seamless extension to deep neural networks will follow with multidisciplinary computational examples.
Learning Outcomes
- Apply deep learning models for real-life problems
- Understand and design deep learning architectures
- Apply the TensorFlow library for practical problems
- Apply machine learning models on edge devices
Course Content
- Essential linear algebra for deep learning
- Fundamentals of linear classification: weights, bias, scores, and loss functions
- Calculus for the gradient descent algorithm
- Forward and backward propagation with regularization
- Batch processing for large datasets
- Linear to nonlinear classification via activation functions
- Computational setup of a shallow neural network
- Tuning neural network performance
- Pre-processing data and batch normalization
- Cross-validation for validating model performance
- Extending the computational setup from a shallow to a deep neural network
- Introduction to the TensorFlow library
- Application projects: implementing shallow and deep neural network models using TensorFlow; implementing machine learning models on edge devices using Edge Impulse.
Pre-requisites
- A strong will to explore and learn new computational ideas
- Prior experience with basic programming using Python/MATLAB/R/C/C++ to the extent of following and understanding pre-filled codes under instructor guidance
Name of the Coordinators
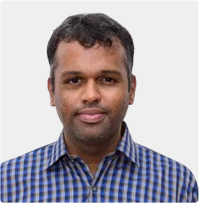
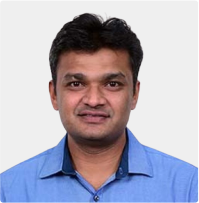
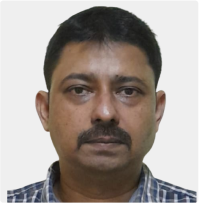
Distinctive Features
- Hands-on and fast-paced introduction to the principles of deep learning using Python
- Solid foundation in the computational components of deep learning
Offered by
Manipal School of Information Sciences, MAHE